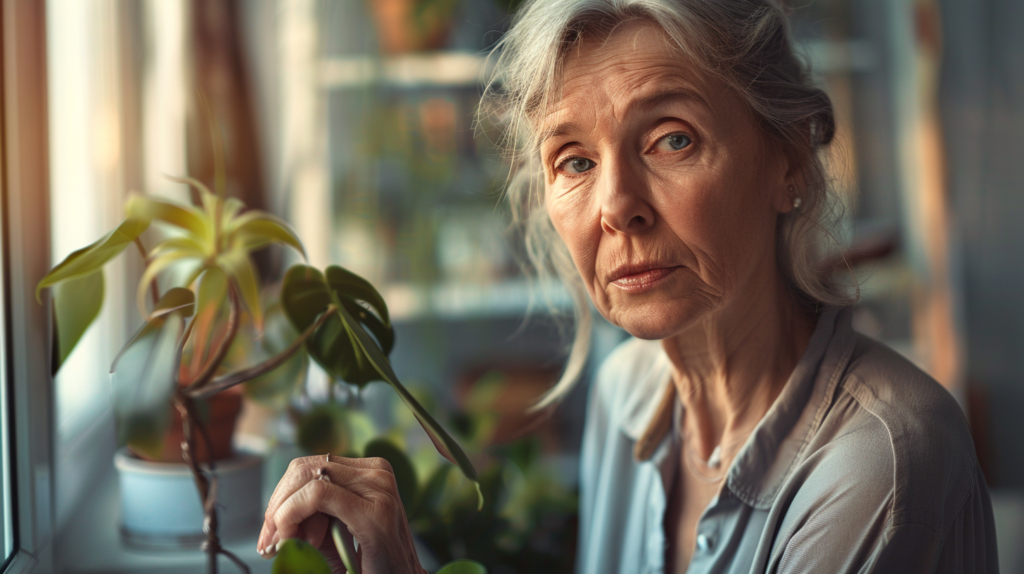
Dr. Marco V. Benavides Sánchez.
Alzheimer’s disease (AD) stands as a formidable challenge in healthcare, affecting millions globally with its progressive cognitive decline. However, recent advancements in technology, particularly in the realms of machine learning and deep learning, offer promising avenues for early detection and intervention. In this article, we delve into how these innovative approaches are reshaping the landscape of Alzheimer’s disease prediction.
Understanding Alzheimer’s Disease
Alzheimer’s disease is a progressive brain disorder that affects memory, thinking skills, and behavior. It is the most common cause of dementia, a general term for memory loss and other cognitive abilities serious enough to interfere with daily life. The disease gradually destroys brain cells, leading to a decline in memory, reasoning, and ultimately, the ability to carry out simple tasks. Symptoms often start slowly and worsen over time, affecting one’s ability to remember recent events, recognize loved ones, or even communicate effectively.
While age is a significant risk factor, Alzheimer’s can also affect younger individuals. Currently, there is no cure for Alzheimer’s disease, but treatments are available to manage symptoms and improve quality of life. Ongoing research aims to uncover more about the disease’s causes, risk factors, and potential treatments. Understanding Alzheimer’s is crucial for early detection, allowing for interventions that can potentially slow down its progression and enhance overall well-being for those affected and their caregivers.
The Role of Machine Learning
Machine learning (ML) algorithms, particularly when applied to neuroimaging data, have shown remarkable potential in predicting the onset and progression of Alzheimer’s disease. Neuroimaging techniques such as Magnetic Resonance Imaging (MRI) and Positron Emission Tomography (PET) provide detailed insights into brain structure and function, which can be leveraged by ML models to identify patterns indicative of Alzheimer’s pathology.
1. Early Detection with Neuroimaging
Researchers have developed ML models that analyze neuroimaging data to predict whether individuals with mild cognitive impairment (MCI) will progress to Alzheimer’s disease dementia. These models integrate various types of data, including MRI scans that highlight structural changes in the brain and PET scans that detect abnormal metabolic activity associated with Alzheimer’s.
Studies have demonstrated that combining multiple modalities of neuroimaging data enhances the accuracy of predictions. For instance, convolutional neural networks (CNNs), a type of deep learning model, have been successful in automatically extracting features from MRI images, enabling more precise identification of Alzheimer’s-related changes compared to traditional methods.
2. Performance and Potential
The performance of ML models in Alzheimer’s prediction is continuously improving. For instance, support vector machines (SVMs) have achieved mean accuracies around 75.4%, while more sophisticated deep learning models like Convolutional Neural Networks (CNNs) have surpassed 78.5% accuracy. These advancements underscore the potential of ML in providing clinicians with robust tools for early diagnosis and personalized treatment planning.
Moreover, integrating genetic, clinical, and cognitive data with neuroimaging further enhances prediction accuracy. This holistic approach not only aids in early detection but also offers insights into the complex interplay of factors contributing to Alzheimer’s disease progression.
3. Challenges and Opportunities
Despite these advancements, several challenges remain. One significant hurdle is the need for large, diverse datasets to train and validate ML models effectively. The availability of such datasets is crucial for developing reliable algorithms capable of generalizing across different populations and healthcare settings.
Additionally, interpreting ML model outputs in clinical practice requires careful consideration. Clinicians must understand the strengths and limitations of these tools to make informed decisions about patient care. Ethical concerns, such as patient privacy and consent for data use, also warrant attention as ML-based diagnostics become more integrated into healthcare systems.
The Future Outlook
Looking ahead, the future of Alzheimer’s disease prediction lies at the intersection of advanced technology and clinical expertise. Ongoing research continues to refine ML algorithms, exploring new avenues such as biomarker discovery and predictive analytics. These efforts aim not only to improve diagnostic accuracy but also to uncover novel insights into disease mechanisms that could inform targeted therapies.
Moreover, the democratization of AI tools and cloud computing allows for broader access to sophisticated diagnostic capabilities, potentially transforming how Alzheimer’s disease is managed globally. Collaborations between researchers, healthcare providers, and technology innovators are pivotal in driving these advancements forward.
Conclusion
Machine learning holds immense promise in revolutionizing Alzheimer’s disease diagnostics. By harnessing the power of neuroimaging and integrating multidimensional data, ML models enable early detection and personalized care strategies. While challenges persist, ongoing research and technological innovations continue to pave the way for a future where Alzheimer’s disease may be detected earlier, treated more effectively, and ultimately, prevented.
As we navigate this evolving landscape, interdisciplinary collaboration and a commitment to ethical AI practices will be essential in maximizing the benefits of machine learning in healthcare. Together, we can strive towards a future where Alzheimer’s disease is no longer a daunting diagnosis, but a condition managed with precision and compassion.
For further reading:
(1) Machine learning methods for predicting progression from mild cognitive…
(2) Machine Learning and Deep Learning Algorithms for Alzheimer Disease ….
(3) A systematic review on machine learning and deep learning techniques in ….
(4) Deep Learning-Based Prediction of Alzheimer’s Disease Using … – MDPI.
(5) Neuroimaging and machine learning for studying the pathways from mild ….
#Emedmultilingua #Tecnomednews #Medmultilingua
Leave a Reply