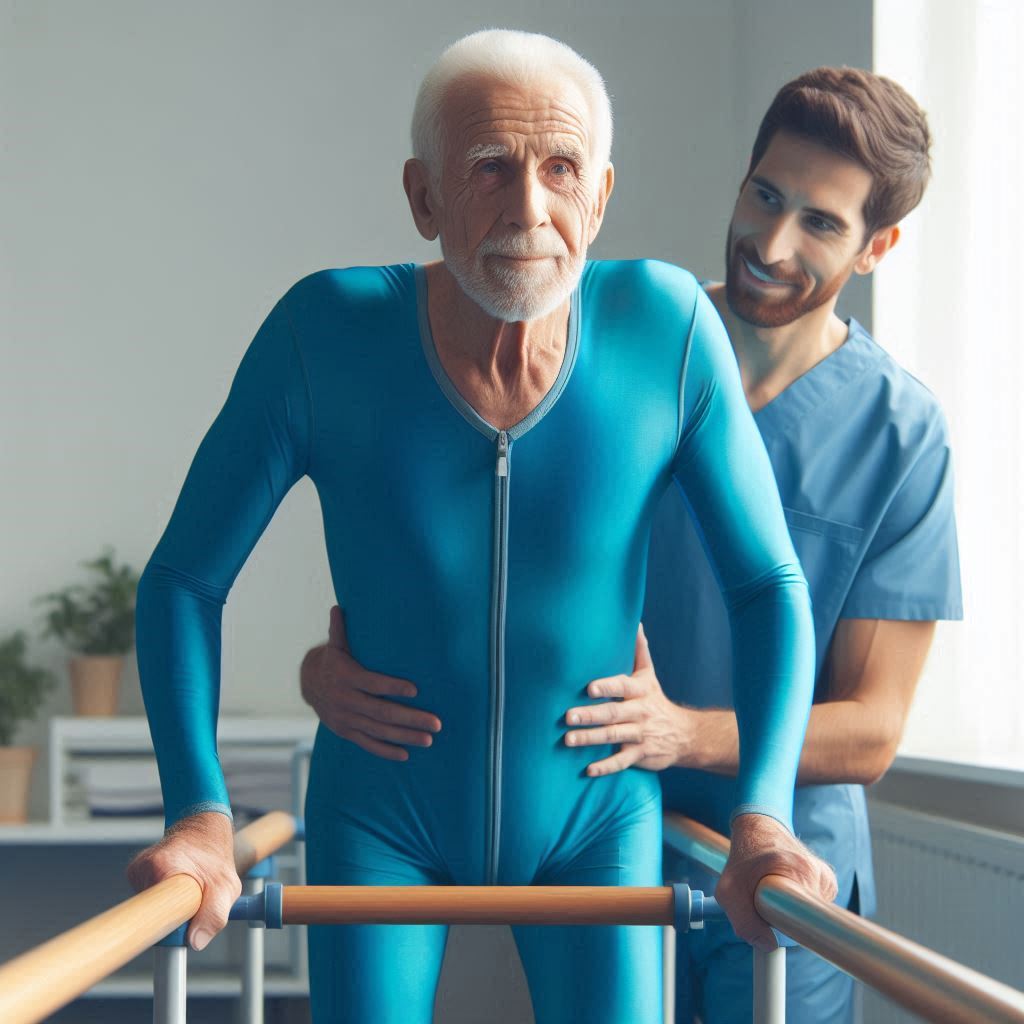
Dr. Marco V. Benavides Sánchez.
Understanding Freezing of Gait (FOG) in Parkinson’s Disease
Parkinson’s disease (PD) is a progressive neurological disorder that affects movement. One of the most challenging symptoms faced by people with advanced-stage Parkinson’s disease is Freezing of Gait (FOG). Imagine feeling like your feet are glued to the floor, unable to take a step forward despite your best efforts. This is what FOG feels like for many individuals with Parkinson’s. The term Freezing of Gait refers to this temporary, involuntary inability to move. These sudden episodes of “freezing” can occur without warning, leading to an increased risk of falls and significantly impacting the quality of life.
The Importance of Predicting FOG
Predicting and preventing FOG is crucial for improving the safety and well-being of Parkinson’s patients. Traditionally, FOG has been managed through medication adjustments and physical therapy, but these approaches are often reactive rather than proactive. The ability to predict FOG episodes in real-time can enable timely interventions, allowing patients to take preventive measures and avoid potentially dangerous falls.
The Promise of Wearable Sensors
Wearable sensors have emerged as a promising tool for monitoring and predicting FOG. These sensors, which can be embedded in shoes or insoles, continuously collect data on various aspects of gait and movement. By analyzing this data, it is possible to detect patterns that indicate an impending FOG episode. However, existing FOG prediction methods have primarily relied on single-sensor systems, such as accelerometers or inertial measurement units [ ] (IMUs). While these systems provide valuable information, they are limited in their ability to capture the full complexity of gait dynamics.
Enter MCT-Net: A Game-Changer in FOG Prediction
To address the limitations of single-sensor systems, researchers have developed the Multi-Channel Time-Series Neural Network (MCT-Net). This innovative approach leverages the power of deep learning to integrate data from multiple sensors, providing a more comprehensive and accurate prediction of FOG episodes. Let’s explore the key features and advantages of MCT-Net.
Key Features of MCT-Net
1. Causal Convolutions: One of the standout features of MCT-Net is its use of causal convolutions. Unlike traditional convolutions that consider data points without regard to their temporal order, causal convolutions respect the sequence of data points. This means that MCT-Net can effectively capture the temporal dynamics of gait, making it well-suited for predicting events like FOG that unfold over time.
2. Intra-Channel and Inter-Channel Transformers: MCT-Net goes beyond simple data integration by employing intra-channel and inter-channel transformers. Intra-channel transformers extract features from individual sensor channels, while inter-channel transformers combine information from different sensor positions. This sophisticated approach helps overcome interference between sensors and ensures that the model captures the most relevant information for accurate predictions.
3. Real-Time Implementation: One of the most exciting aspects of MCT-Net is its real-time implementation. This means that the model can operate on wearable devices, continuously analyzing sensor data and providing timely alerts to patients. For example, if MCT-Net detects that a FOG episode is imminent, it can send an alert to the patient’s smartphone or wearable device, allowing them to take preventive actions.
The Impressive Performance of MCT-Net
The performance of MCT-Net in predicting FOG is nothing short of remarkable. Compared to other state-of-the-art FOG prediction methods, MCT-Net consistently outperforms in terms of accuracy and F1-score. Let’s take a closer look at the results:
– Accuracy: MCT-Net achieves an impressive 96.21% accuracy in predicting FOG episodes. This high level of accuracy means that the model can reliably identify when a FOG episode is about to occur.
– F1-Score: The F1-score is a measure of a model’s precision and recall. MCT-Net attains an average F1-score of 80.46%, indicating a good balance between correctly identifying FOG episodes and minimizing false positives.
– Prediction Time: Perhaps most importantly, MCT-Net can predict FOG occurrences approximately 2 seconds before they happen. While this may seem like a short window, even a brief advance warning can be invaluable for patients, giving them the chance to stabilize themselves and avoid a fall.
Real-World Impact and Future Directions
The real-world impact of MCT-Net has the potential to be transformative for individuals living with Parkinson’s disease. By providing timely alerts and allowing for proactive interventions, MCT-Net can significantly reduce the risk of falls and improve the overall quality of life for patients. Moreover, the integration of MCT-Net into wearable devices means that this technology can be seamlessly incorporated into daily life, providing continuous support and monitoring.
However, while MCT-Net shows great promise, there is still room for further research and development. Expanding the dataset to include a more diverse population of individuals experiencing FOG will help enhance the model’s robustness and generalizability. Additionally, exploring ways to optimize the model for different types of wearable sensors and refining the real-time implementation will be important steps in bringing this technology to a broader audience.
The Broader Context: AI and Healthcare
The development of MCT-Net is part of a broader trend of leveraging artificial intelligence (AI) to improve healthcare outcomes. AI has the potential to revolutionize the way we diagnose, monitor, and treat a wide range of medical conditions. In the context of Parkinson’s disease, AI-driven approaches like MCT-Net can provide personalized and adaptive care, tailored to the unique needs of each patient.
The integration of AI into healthcare is not without challenges. Ensuring the accuracy and reliability of AI models, protecting patient privacy, and addressing ethical considerations are all important factors that need to be carefully managed. However, the potential benefits of AI in healthcare are immense, and the development of innovative solutions like MCT-Net represents a significant step forward.
Conclusion
The prediction and prevention of Freezing of Gait in Parkinson’s disease is a critical area of research with the potential to significantly improve the lives of patients. The Multi-Channel Time-Series Neural Network (MCT-Net) represents a groundbreaking approach to FOG prediction, leveraging the power of deep learning and wearable sensors to provide accurate, real-time alerts. With its impressive performance and potential for real-world impact, MCT-Net is poised to become a valuable tool in the management of Parkinson’s disease.
As we continue to explore the possibilities of AI in healthcare, the development of innovative solutions like MCT-Net offers a glimpse into a future where technology plays a central role in improving patient outcomes and quality of life. For individuals living with Parkinson’s disease, this future holds the promise of greater independence, safety, and well-being.
For further reading:
(3) Feature Learning for Detection and Prediction of Freezing of Gait in Parkinson’s Disease.
(4) Wearable-Sensor-Based Detection and Prediction of Freezing of Gait in Parkinson’s Disease: A Review.
(5) Detection and prediction of freezing of gait with wearable sensors in Parkinson’s disease.
(6) What is an Inertial Measurement Unit? · VectorNav.
#Tecnomednews #Emedmultilingua #Medmultilingua
Leave a Reply