
Dr. Marco V. Benavides Sánchez.
The advent of artificial intelligence (AI) in the field of medical imaging is marking an unprecedented revolution in modern medicine. What once seemed the exclusive domain of highly specialized physicians is now on the verge of being complemented, and even surpassed, by advanced AI algorithms.
This technological leap not only promises an increase in diagnostic accuracy, but also a complete redesign of workflows in hospitals and clinics, optimizing time and resources in a way that conventional medicine has never before achieved.
Data Collection and Annotation: The First Step Towards Accuracy
The process begins with data collection, a task that has been, and will continue to be, the backbone of AI’s success in medicine. Without a robust database, there is no algorithm that can function effectively. In imaging, this involves collecting millions of medical images, from MRIs to CT scans, which must be meticulously annotated by medical experts.
This is where the value of the human specialist remains indispensable: manual annotation is key for the algorithm to learn to differentiate between a benign condition and a serious pathology. In addition, data diversity is crucial. Databases must include images from patients of different ethnicities, genders, ages, and morphologies to avoid bias and ensure the applicability of algorithms globally.
Preprocessing: The Basis for Consistent Analysis
Before algorithms can even begin to analyze these images, they must be preprocessed. Preprocessing includes several steps, such as normalizing pixel values, removing noise and artifacts, and, in many cases, using data augmentation techniques.
These techniques are essential to make algorithms more robust against natural variations in images, such as different capture angles or varying quality. At this point, advances in preprocessing are enabling AI algorithms to surpass human ability to detect subtle patterns that could be early-stage disease signals.
Algorithm Choice and Training: The Heart of the Process
The real driving force behind AI success in imaging lies in choosing the right algorithm and then training it. Convolutional neural networks (CNNs), which are specifically designed for processing visual data, are the most widely used in this field. These networks mimic the functioning of the human brain when analyzing images, allowing for the identification of patterns and details that might escape the human eye, even that of an expert.
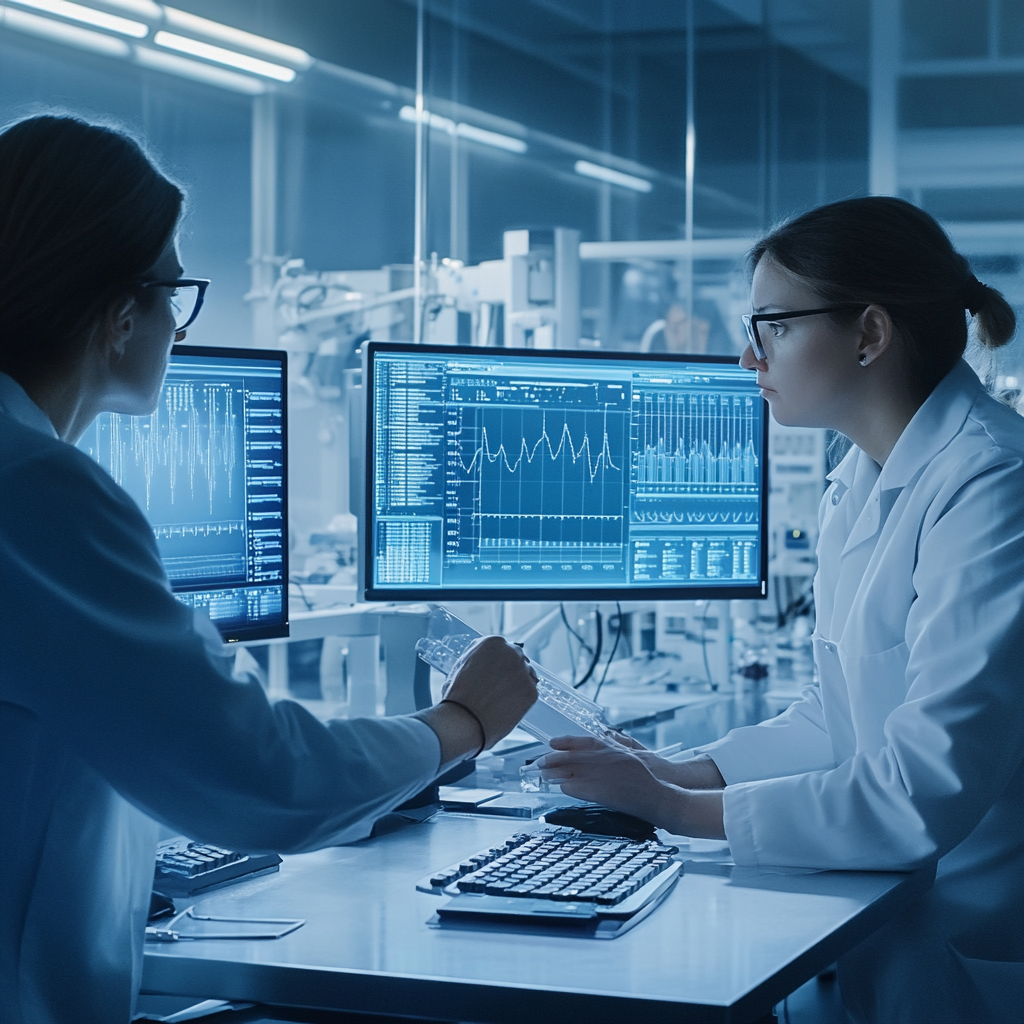
However, training these networks is not a trivial task. It requires not only large amounts of data, but also constant supervision to ensure that the algorithm learns correctly. Errors at this stage could result in misdiagnoses, underlining the importance of human involvement throughout the process.
Furthermore, there is a growing trend towards the use of multimodal AI models, which integrate imaging data with other types of clinical information, such as electronic medical records or laboratory tests. These approaches not only increase diagnostic accuracy, but also allow for more personalized and effective treatment for each patient.
Validation, Fine-Tuning, and Deployment: Towards Clinical Implementation
Once the algorithms have been trained, the next step is rigorous validation, which ensures that the model works correctly in real-world situations. Testing on independent data sets, cross-validation, and fine-tuning are essential to ensure that the models not only work in controlled environments, but are applicable in daily clinical practice.
This process can be long and expensive, but it is a necessary step before AI algorithms can be safely deployed in hospitals and clinics. We cannot allow undetected errors to affect the lives of patients, so the validation phase must be treated as seriously as any clinical trial.
Ethical and Regulatory Considerations: Not Everything is Technology
The use of AI in medicine raises ethical dilemmas and regulatory challenges that cannot be ignored. Access to patient data, equity in diagnosis and treatment, as well as transparency in how algorithms work, are issues of great relevance. AI should not be seen only as a technical tool, but as an extension of the medical act, which means that it must be governed by the same ethical principles than traditional medicine.
For example, what happens if an algorithm makes a mistake and affects a patient’s life? Who is responsible? These are questions that developers and regulators must address before AI is fully integrated into the healthcare system.
In addition, it is crucial that patients understand how AI is involved in their healthcare. Trust in technology is as important as its accuracy, and a lack of transparency could erode that trust. Here, effective communication between healthcare professionals and patients will play a critical role in the success of AI implementation.
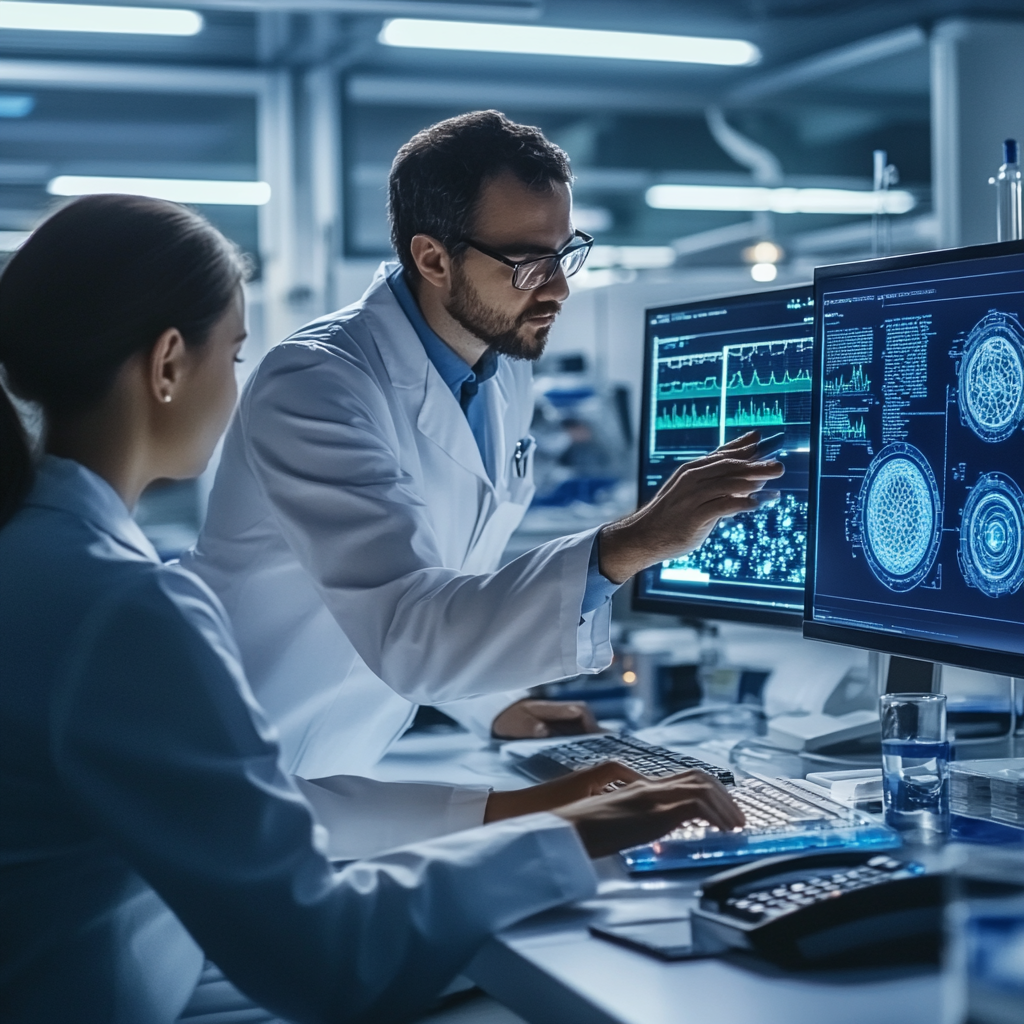
The Future: AI as a Complement, Not a Substitute
Although AI has proven to be a powerful tool in medical imaging, it is still far from replacing human professionals. Its true value lies in complementing the skills of doctors, helping them make faster and more accurate diagnoses, and ultimately improving outcomes for patients. Nonetheless, enthusiasm must be accompanied by caution. AI is only as good as the data it receives and the human decisions that shape it.
In conclusion, artificial intelligence is shaping a future where medical diagnosis will be more efficient and accessible than ever before. But for this promise to be fulfilled, we must ensure that the technology is used responsibly, with a clear ethical approach and constant oversight. The potential is immense, but it is our duty as a society and as physicians to ensure that its impact is truly positive for all.
To learn more:
(1) AI and machine learning in medical imaging: key points from development to translation
(3) A framework to integrate artificial intelligence training into radiology residency programs
(4) Redefining Radiology: A Review of Artificial Intelligence Integration in Medical Imaging
#Emedmultilingua #Tecnomednews #Medmultilingua
Leave a Reply